Systems Medicine
Understanding Disease Pathophysiology at the Molecular Level
The Clinical Epidemiology and Systems Medicine (CESM) uses a combination of modern systems medicine methods and fundamental concepts from clinical epidemiology to gain new vantage points on complex disease. Data from all biological levels — from DNA, to the transcriptome, proteome and metabolome, as well as regulatory layers including DNA methylation — are holistically integrated with detailed clinical phenotype data to realistically depict the biological system in context of the disease of interest. A transdisciplinary team of experts works together to understand if and how these processes are causally related to the disease.Sequential Multi-Omics Data
The Gutenberg cohorts managed by the CESM (e.g. Gutenberg Health Study, Gutenberg COVID-19 Study, MyoVasc) all share the feature that biomaterial is sequentially collected at several time points. Multi-omics profiling is conducted on biosamples and post-processing performed according to highly standardized procedures. This provides a high-resolution window into disease pathophysiology at all stages — from pre-disease to acute disease, its progression and resolution.Advancing Analytic Methods to Promote Knowledge Generation
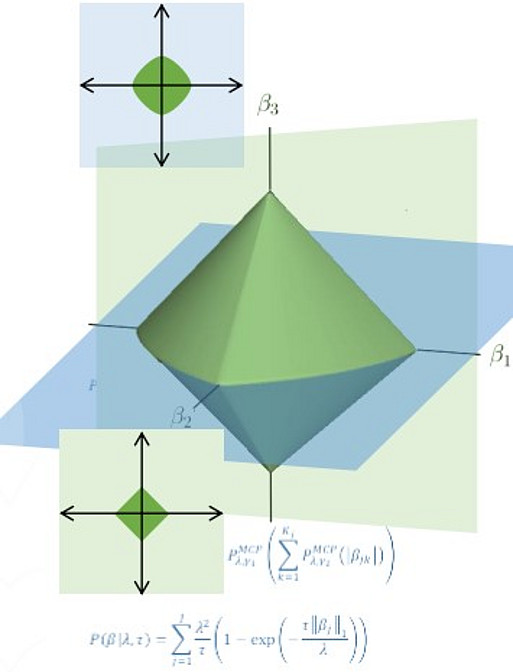
To support innovative systems medicine research, the CESM employs statisticians and machine learning researchers who are dedicated to the development of new biostatistical and machine learning methods. These methods are optimized to the specific purpose of each investigation, whether it be drug target discovery, mechanistic explorations of pathway involvement, monitoring disease development over time, or integrative multi-omics approaches including less well-characterized data levels such as the lipidome.
In all applications, it is important that key features of the biological system under investigation are adequately captured, such as non-linear relationships, interactions, and grouping structures (e.g. based on shared pathways, functions or sequence homology). To accommodate these needs in extremely high-dimensional settings, new methods are continuously proposed and implemented.
In all applications, it is important that key features of the biological system under investigation are adequately captured, such as non-linear relationships, interactions, and grouping structures (e.g. based on shared pathways, functions or sequence homology). To accommodate these needs in extremely high-dimensional settings, new methods are continuously proposed and implemented.
Deep Learning for Enhanced Feature Extraction from Clinical Imaging
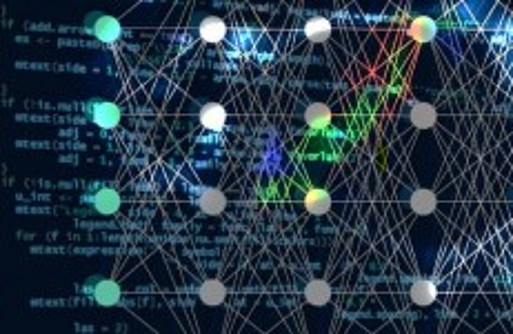
A large amount of clinical imaging data is produced on a daily basis in clinics. Despite recent progress, only a fraction of this data is currently leveraged for clinical or research applications. New developments in artificial intelligence (AI), especially in the subfield of deep learning, open up the possibility of exploiting the large potential of this untapped resource. CESM researchers and affiliates work together to optimize deep learning architectures to automate and improve clinical imaging pipelines as well as to extract novel features that could enhance and accelerate individualized risk prediction.